Computer Numerical Control (CNC) machining is a manufacturing process in which pre-programmed computer software dictates the movement of factory tools and machinery. The process is widely used in manufacturing for producing precise components with a high degree of accuracy. Traditionally, CNC machining strategies have been developed by skilled machinists who rely on their experience and knowledge to optimize the process. However, the advent of artificial intelligence (AI) has introduced new opportunities to automate and optimize these strategies, significantly improving efficiency, reducing waste, and enhancing the overall quality of manufactured parts.

AI CNC Machining Overview
CNC machining involves the use of computers to control machine tools such as lathes, mills, routers, and grinders. The process starts with the creation of a digital model, which is then converted into a set of instructions, often referred to as G-code, that the machine follows to cut and shape the material.
CNC machines are capable of producing complex shapes with high precision and are used in various industries, including aerospace, automotive, medical devices, and electronics.
Traditional Strategy Development
In traditional CNC machining, the development of machining strategies involves determining the optimal tool paths, cutting speeds, feed rates, and other parameters that affect the machining process. This task is typically performed by experienced machinists who use a combination of empirical data, trial-and-error, and simulation software to optimize the process. The goal is to maximize the efficiency of the machining operation while minimizing tool wear, material waste, and production time.
Challenges in Traditional Strategy Development
The traditional approach to CNC machining strategy development presents several challenges. First, it is time-consuming and labor-intensive, requiring significant expertise and experience. Second, the optimization process is often iterative, involving multiple rounds of testing and adjustment to achieve the desired results. Third, the complexity of modern components, which often have intricate geometries and tight tolerances, makes it difficult to manually develop optimal strategies. Finally, human error and variability in decision-making can lead to inconsistencies in the quality of the machined parts.
The Role of Artificial Intelligence in CNC Machining
AI has the potential to revolutionize CNC machining by automating the generation of machining strategies and optimizing them in real time. AI-based systems can analyze vast amounts of data, learn from previous machining operations, and make decisions that optimize the process for specific goals, such as minimizing production time, reducing tool wear, or improving surface finish. The integration of AI into CNC machining can be achieved through various techniques, including machine learning, neural networks, and genetic algorithms.
Machine Learning in CNC Machining
Machine learning (ML) is a subset of AI that enables computers to learn from data and make predictions or decisions without being explicitly programmed. In the context of CNC machining, ML algorithms can be trained on historical data from previous machining operations, including parameters such as tool paths, cutting speeds, feed rates, and resulting part quality. By analyzing this data, the ML model can identify patterns and correlations that can be used to predict the optimal machining parameters for new parts.
For example, an ML model can be used to predict the optimal tool path for a given geometry, taking into account factors such as material properties, machine capabilities, and desired surface finish. The model can also adjust parameters in real-time based on feedback from the machine, such as tool wear or vibrations, to ensure consistent quality and reduce the likelihood of defects.
Neural Networks in CNC Machining
Neural networks are a type of machine learning algorithm inspired by the human brain’s structure and function. They consist of interconnected nodes, or neurons, that process and transmit information. Neural networks are particularly well-suited for tasks involving pattern recognition, classification, and prediction, making them a valuable tool in CNC machining.
In CNC machining, neural networks can be used to optimize tool paths, predict tool wear, and detect anomalies in the machining process. For instance, a neural network can be trained to recognize patterns in the vibration data collected from a machine during operation. If the network detects an anomaly, such as an increase in vibration that could indicate tool wear or a potential defect, it can alert the operator or automatically adjust the machining parameters to compensate.
Genetic Algorithms in CNC Machining
Genetic algorithms (GAs) are another AI technique that can be used to optimize CNC machining strategies. GAs are inspired by the process of natural selection, where the fittest individuals are selected for reproduction to produce the next generation. In the context of CNC machining, a GA can be used to explore a wide range of possible machining strategies and select the ones that perform the best according to predefined criteria.
The GA starts with a population of random machining strategies, each represented by a set of parameters such as tool paths, cutting speeds, and feed rates. The strategies are evaluated based on their performance, and the best-performing ones are selected for reproduction. The selected strategies are then combined and mutated to create a new generation of strategies, which are again evaluated and selected. This process is repeated until the GA converges on an optimal or near-optimal machining strategy.
Applications of AI in CNC Machining
AI can be applied to various aspects of CNC machining, including tool path optimization, process monitoring, and predictive maintenance.
- Tool Path Optimization: AI can generate optimal tool paths that minimize machining time and improve surface finish. By analyzing the geometry of the part and the capabilities of the machine, AI algorithms can create tool paths that reduce the number of passes, minimize tool changes, and avoid collisions.
- Process Monitoring: AI can be used to monitor the machining process in real time, analyzing data from sensors such as accelerometers, microphones, and thermocouples. This data can be used to detect anomalies, predict tool wear, and prevent defects. AI-based systems can also adjust machining parameters on the fly to maintain consistent quality and extend tool life.
- Predictive Maintenance: AI can predict when a machine or tool is likely to fail, allowing for preventive maintenance to be scheduled before a breakdown occurs. By analyzing historical data on machine performance and maintenance records, AI algorithms can identify patterns that indicate impending failures. This can help reduce downtime, extend the life of equipment, and lower maintenance costs.
- Material Optimization: AI can also assist in selecting the best materials for a given machining operation. By analyzing material properties, cost, and availability, AI can recommend materials that will optimize the machining process and meet the desired specifications.
Challenges and Future Directions
While AI has the potential to transform CNC machining, several challenges must be addressed to fully realize its benefits. One challenge is the need for large amounts of high-quality data to train AI models. In many cases, data from previous machining operations may be incomplete, inconsistent, or unavailable, making it difficult to develop accurate models. Another challenge is the integration of AI into existing manufacturing systems, which may require significant changes to workflows, software, and hardware.
Moreover, there is a need for standardization in AI applications for CNC machining to ensure compatibility and interoperability between different systems and vendors. The lack of standardization can hinder the adoption of AI technologies and limit their effectiveness.
Despite these challenges, the future of AI in CNC machining is promising. Advances in machine learning, neural networks, and other AI technologies are expected to continue improving the efficiency, accuracy, and flexibility of CNC machining. As AI becomes more integrated into manufacturing processes, it has the potential to revolutionize the industry, enabling the production of increasingly complex and high-quality components with greater efficiency and lower costs.
Conclusion
The integration of AI into CNC machining represents a significant advancement in manufacturing technology. By automating the generation and optimization of machining strategies, AI can overcome many of the limitations of traditional methods, leading to improved efficiency, reduced waste, and enhanced quality. As AI technologies continue to evolve, their application in CNC machining is likely to expand, offering new opportunities for innovation and competitiveness in the manufacturing industry.
The Detail Of BE-CU cnc machining Shop
BE-CU.COM – As an accomplished CNC machining Service Manufacturer and CNC shop, BE-CU Prototype has been specialized in OEM CNC lathing, custom CNC machining parts production and rapid CNC machining services China for over 35 years and always maintaining the highest standard in delivery speed and reliable quality of precision CNC manufacturing components. With the help of high-level technology and efficient equipment, as well as rigorous attitude, BE-CU passed the ISO9001:2015 quality certification, which supports the long-term development of CNC milling services, CNC turning services, CNC milling-turning, CNC drilling services, 3/4/5 axis machining, gear machining services, CNC machining China custom parts and service, small parts machining, etc.Our CNC machining products can be utilized in a broad range of industries. Contact us for email: [email protected]
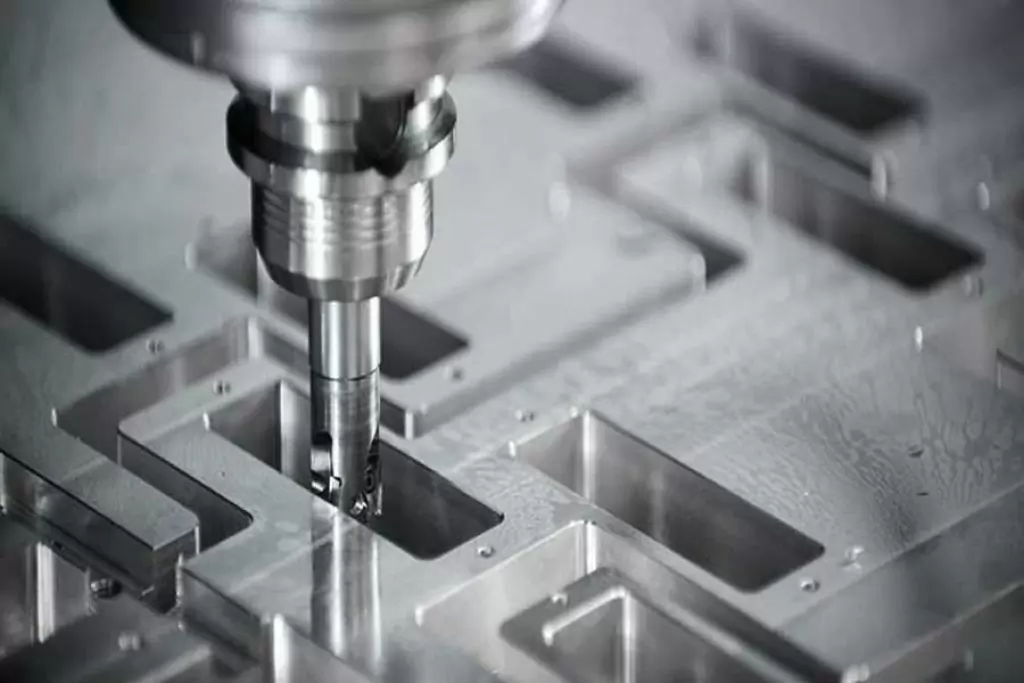